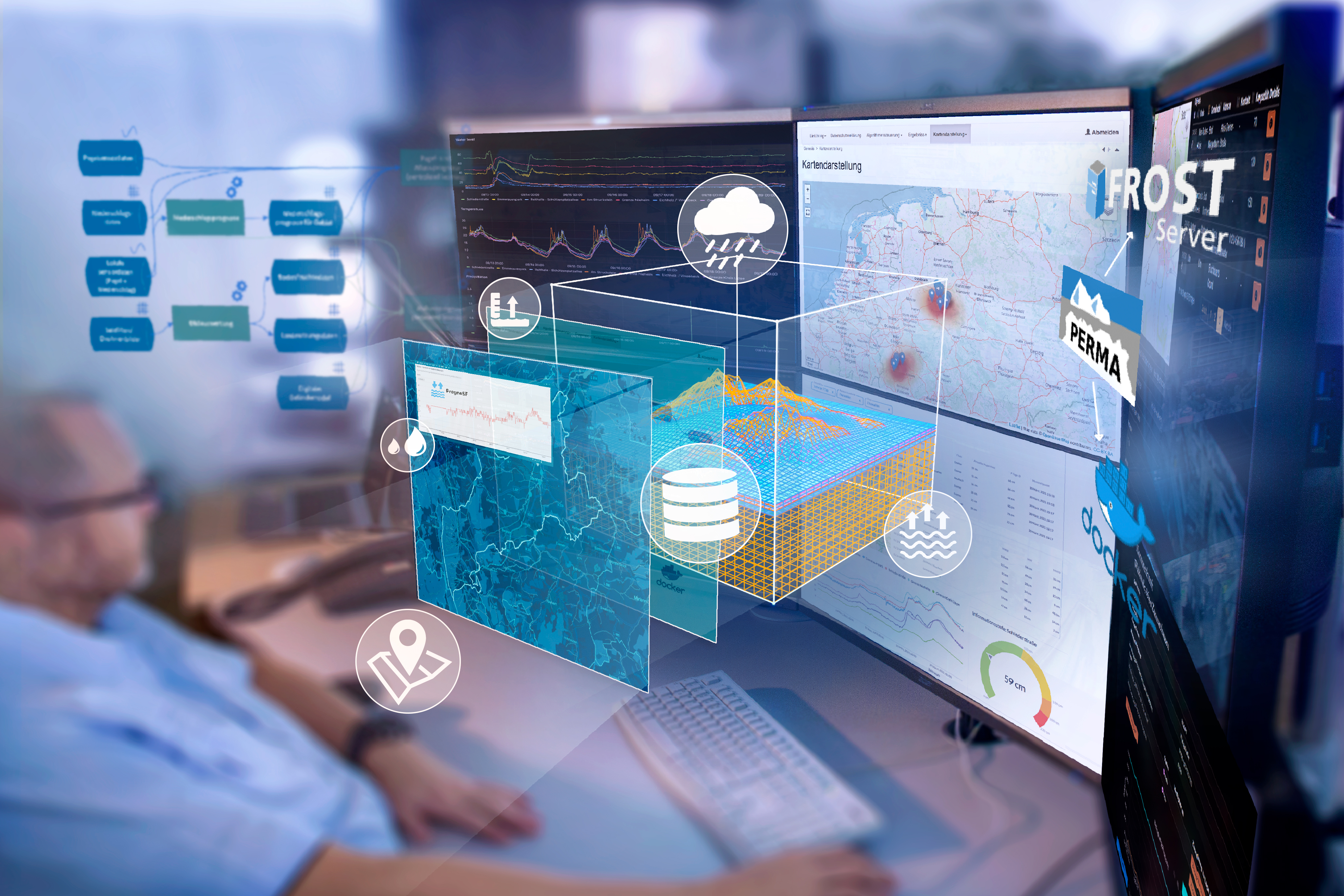
Brief description of the project
Due to climate change, growing urban areas and more and more impermeable surfaces, floods triggered by intense rainfall are becoming more frequent and severe. Although early warning systems have been the subject of research for a long time, predictions, especially for local events, are highly inaccurate.
In our in-house PrognoSF project, we tackle this problem with the aim of making disaster prevention and management more effective. The idea is to combine sensor data on precipitation, water levels, and land use, obtained from remote sensing or local Smart city platforms, with high-resolution precipitation forecasts provided by the German National Meteorological Service (DWD).
To this end, we connect sensors and existing data platforms with a modern sensor data management system and AI modules, relying heavily on open standards. In particular, we use our own open-source implementation of the OGC SensorThings API (aka the FROST® server), with PERMA allowing for easy integration of AI calculation modules via Docker Containers, and our WebGenesis® decision support web platform.
Development objectives in detail
Our development activities in PrognoSF include designing specific sensor stations and connecting them to smart city platforms via LoRaWAN, , as well as developing various AI-based modules with the aim of enabling faster and more accurate predictions for improved early warning. The modules, first versions of which have been implemented, include a local precipitation forecast, image processing that extracts current land use from satellite imagery, and water level and discharge forecasting based on historical data, real-time sensor readings, and weather.
Current status
PrognoSF is currently (2024) being evaluated in workshops with civil protection authorities and end users to examine the advantages and disadvantages compared to existing systems. Our aim is to provide the authorities with a practical tool that extends the warning time and predicts risk areas more accurately.