
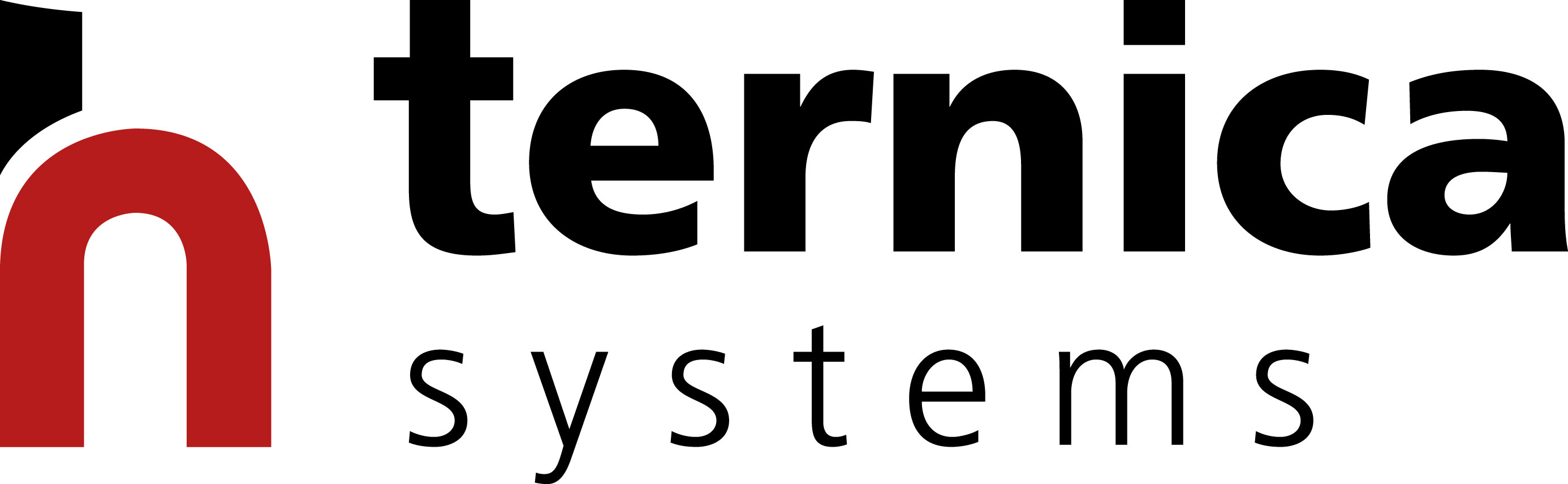
Initial situation
»Vehicle Make and Model Recognition« (VMMR) - the fine-grained recognition of vehicle makes, models, years of manufacture and paint colors in image data - is used to recognize vehicles even in cases where the license plate is not clearly recognizable or potentially falsified.
Using modern AI methods, hundreds of vehicle models can be classified in video data in real time or recognized in specific search queries. However, a particular challenge, especially for critical applications, is the accuracy and timeliness of the data sets used to train the classification process: Teaching vehicles requires high-quality training data in large quantities. The collection of real data, which is annotated by humans, is error-prone, data protection-critical and involves a great deal of effort. Accordingly, current vehicle models are only reliably recognized late in current approaches.